6 The Economics of Discrimination
Caroline Krafft
What is discrimination?
Discrimination is the unjust or unequal treatment of an individual or group based on a specific characteristic, such as their race, age, or gender identity. In the United States, a number of laws forbid discrimination on the basis of age, disability, national origin, pregnancy, race/color, religion, or sex.[1] Globally, the United Nations (UN) has passed conventions on eliminating all forms of racial discrimination and discrimination against women.[2]
Although the definition seems straightforward, identifying when an individual or entity is discriminating in practice is quite challenging. This difficulty is because disparities (differences in outcomes) may be the result of current or past discrimination. The fact that women earn 84 cents for every dollar men earn[3] may reflect employers’ discrimination in setting wages, but may also reflect the fact that women choose different majors, or are more likely to take time out of the workforce to care for children. Of course, that women choose different majors may also reflect discrimination in human capital accumulation.
Likewise, the fact that Black men have a one in three lifetime likelihood of imprisonment, while white men have a one in seventeen chance[4] may be due to a variety of factors, such as historical housing discrimination and poor local labor market opportunities, as well as discrimination in the criminal justice system.[5] Identifying the source of disparities—for instance in the case of imprisonment, whether disparities are due to unequal and discriminatory outcomes around education, employment, or poverty as factors in committing crimes, or in unequal chances of arrests, convictions, or sentences—is critical to addressing and reducing these disparities.
Causes of discrimination
Discriminatory “tastes”
Economists have two main theories concerning the causes of discrimination. The first theory is that individuals have “tastes” or preferences for discrimination.[6] This taste-driven discrimination theory suggests that factors such as social and physical distance and relative socioeconomic status contribute to tastes for discrimination. Contact with a minority group and the size of a “minority” group matter as well (the minority in this case could actually be a majority that has historically been disempowered, e.g., women).[7] Tastes for discrimination mean that individuals are effectively willing to forfeit income to avoid certain transactions or interactions. For instance, landlords may prefer to rent only to individuals of a certain race or religion, even though they could charge higher rents if they opened up to a broader market.
Statistical discrimination
The second theory is statistical discrimination, which assumes discrimination is essentially an information problem.[8] For instance, in the labor market, employers may have imperfect information about the productivity of individual workers. Consider the case of an employer hiring a new carpenter for a construction company. The employer has information from applicants’ resumes on their education, training and past work experience. She can even administer a test to prospective employees to measure their skills, perhaps building a stair rail. The resume information and the test are, however, imperfect signals of the employee’s productivity. This uncertainty and imperfect information cause the employer to take into account another factor: she believes that women are, on average, less productive carpenters than men. This potentially erroneous statistic, combined with the inability of the resume and skills test to fully signal productivity, will lead the employer to conclude that a man is likely to be more productive than an equally qualified woman. The job is then offered to the man instead of the woman.
A “taste” for discrimination and statistical discrimination are often framed as competing theories. However, as we will see in discussing the empirical studies on discrimination below, there is substantial evidence for each theory. One way of reconciling the theories may be to think of taste-driven discrimination as a potential source of assumptions about individuals’ productivity in the face of incomplete information. Additionally, some individuals may change their assumptions in the face of additional evidence, a case which supports the existence of statistical discrimination. Others with more deeply ingrained prejudices would not reevaluate their assumptions, which lends credence to taste-driven discrimination. Different assumptions regarding individuals and groups may be more or less swayed by information.
Box 6.1: Economists in Action: Lisa Cook Studies Competition and Discrimination[9]
Dr. Lisa D. Cook is a Professor of Economics and International Relations, currently serving on the Board of Governors for the Federal Reserve. She researches economic growth and development, along with financial institutions, innovation, and economic history. She was a Senior Economist for the Council of Economic Advisors, serving in the White House, and was elected to the board of the American Economic Association (AEA). She directed the AEA Summer Training Program, which increases diversity in the field of economics by preparing undergraduate students for graduate degrees in economics.
One important area of Dr. Cook’s research is creating and analyzing data on discrimination, including gathering data on Jim Crow era firms that were friendly towards African Americans, as well as the creation of a national lynching database. In one of her papers Dr. Cook examines the determinants of firms’ discrimination towards potential consumers during the Jim Crow era and prior to the Civil Rights Act. She shows that firm owners segregated and discriminated against African Americans based on white consumers’ discrimination, a case of taste-based discrimination. Reductions in the number of white consumers reduced discrimination and activism among African Americans also helped.
Labor market models of discrimination and its consequences
Regardless of whether discrimination is taste-driven or caused by statistical discrimination, it can essentially be modeled the same way. The approaches to reducing discrimination will be quite different, but the models for the impact of discrimination will be nearly identical. Consider discrimination for the case of the labor market. Recall that employers’ demand for labor is based on productivity. We are now going to name that productivity the marginal revenue product of workers, how much revenue they create for their employers through their work. Operating under statistical discrimination, productivity is assumed to be lower for certain groups.[10] In the case of gender discrimination, employers may assume the marginal revenue product of women is lower because they disproportionately undertake caregiving responsibilities (a case of statistical discrimination). Alternatively, with taste-based discrimination, hiring a less-preferred group imposes a “cost” on employers, effectively modeled as a decrease in the marginal revenue product.
Figure 1 shows discrimination in the labor market for men and women. To simplify, we assume the same labor supply for men and women. However, the demand, which is equal to the marginal revenue product (MRP) is assumed to be lower for women than for men (discrimination). As a result, the equilibrium outcome is that fewer women are employed and women are earning lower wages than men. Although we focus on models of the labor market here, similar ideas apply to other markets. Housing is another example. A landlord might discriminate in supplying housing to individuals with a different religion than his own. This would shift the supply of housing to differentiate between religious groups, with a higher cost (reduced supply) for those of a different religion.
In all cases, there is a substantial challenge when it comes to proving discrimination. It is difficult to measure the MRP of different workers. This then makes it difficult to distinguish between cases where individuals actually are differentially productive on average, such as workers with more training or experience, and when discrimination is occurring.
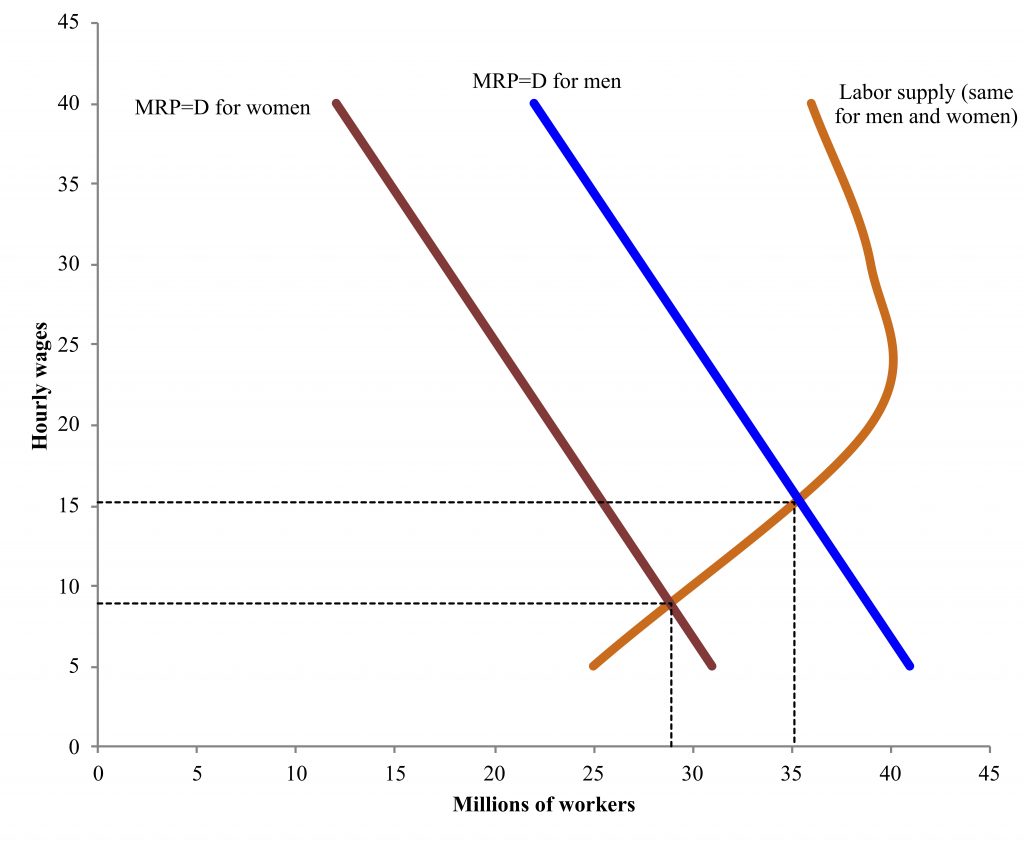
Evidence on Discrimination
There are a variety of forms of discrimination and different groups that are discriminated against globally. This section presents just some of the evidence on discrimination, primarily from the U.S., but from other global contexts as well. Economists rely on a host of different techniques to gather evidence on discrimination. One is multiple regression, also called multivariate regression, a statistical technique where economists try to account for differences in observable characteristics. When checking for wage discrimination, for example, these characteristics would include occupation and education. The remainder of the differences in outcomes would then be attributed to discrimination.
Conducting experiments is another method to assess discrimination. Economists can randomize resumes with different characteristics to apply to jobs or randomize the economic equivalent of “mystery shoppers” with different characteristics to apply for housing. These experiments are typically referred to as audit studies. Experiments are the most effective for being certain about cause and effect, but can be challenging to implement and much more expensive than analyzing existing data with multiple regression. This section presents evidence from both multiple regression models and audit studies on discrimination in education, housing, the labor market, and the criminal justice system.
In education
Discrimination in the education system leads to disparate human capital outcomes that also contribute to labor market disparities.[11] Teachers play a key role in education and their discriminatory attitudes can affect students in a variety of ways. For instance, one experiment demonstrated that teachers gave worse grades and lower secondary school recommendations when assignments (essays) had minority (Turkish) names.[12] Teachers also have lower expectations and negative attitudes that affect their behavior towards minority students, which may in turn affect those students’ performance.[13] Gender bias may be particularly important for Science, Technology, Engineering, and Math (STEM) fields. Science faculty presented with otherwise identical student resumes bearing either a female or male name rated women as less competent than men. Faculty were less likely to hire women, offered a lower salary, and were less likely to mentor women.[14]
In housing
Housing was one of the areas where discrimination in the United States was first measured effectively. Fair housing audits were developed by housing organizations to identify racial discrimination in housing opportunities after the passage of the Civil Rights Act.[15] For example, in assessing Black-white housing disparities, an audit will send two auditors to a housing agent, one white and one Black, for a random sample of advertised housing units. When individuals receive differential treatment, specifically in different offers of housing, and this treatment depends on their race, the audit indicates discrimination. Historically, as of 1981, Black housing seekers were told about 30% fewer available housing units than whites.[16]
More recent studies have taken advantage of the power of the internet; an experiment in the U.S. rental apartment market varied first names, using those commonly associated with whites and African Americans. In some cases, it also included information about credit history and smoking. African-American sounding names had a 9.3 percentage point lower positive response rate than applicants with white-sounding names, indicating discrimination. The additional information on credit history and smoking did differentially affect the gap in response rates, indicating that information and statistical discrimination contributed to disparities.[17] In India, a study using India’s largest real estate website showed that, while an upper-caste Hindu had a 35% chance of a response to a housing application, this was only 22% for a Muslim applicant.[18] An experiment in Sweden varied distinctive ethnic and gender names in applying for rental housing. Arabic/Muslim names received fewer responses than the Swedish male names, and Swedish female names had an easier time accessing housing than Swedish male names.[19] In addition to long-term rentals, these disparities extend to short-term rentals, such as Airbnb vacation rentals. Applications from guests with African-American names were 16% less likely to be accepted relative to otherwise identical guests with distinctively white names.[20] Discrimination also occurs against Airbnb ethnic minority hosts.[21]
In the labor market
Discrimination in the labor market manifests in substantial hiring disparities by race, ethnicity, gender, and disability status. A study sending fake resumes to help-wanted ads in Boston and Chicago found that white names received 50% more callbacks for interviews than African-American names.[22] A similar study in New York City found that Black applicants were half as likely to receive a callback or job offer than white applicants.[23] In interviews for waitstaff jobs in Philadelphia, job applications from women had a 40 percentage point lower chance of receiving a job offer from high-price (and high earning) restaurants than men, in part embodying customer discrimination.[24] An experiment that randomized disclosure of disability status found disability halved the chances of a callback.[25]
In Toronto, a study demonstrated that individuals with foreign experience or with Indian, Pakistani, Chinese, and Greek names were less likely to be hired than those with English names.[26] In Germany, which has a substantial number of Muslim migrants, especially from Turkey, it is common for applicants to send photos with resumes. A study of female applicants that randomized German names, Turkish names, and whether the migrant was wearing a headscarf found significant discrimination against Turkish names and more so against women wearing a headscarf. This discrimination is so pronounced that a female applicant who wears a headscarf and who has a Turkish name would have to send 4.5 times as many applications to receive the same number of callbacks as a woman with a German name and no headscarf.[27]
In the United States, women’s pay is, as of 2023, 84% of men’s pay.[28] Notably, women and men are approaching convergence in their pay at the start of their careers. Figure 6.2[29] shows median weekly earnings by sex, as well as the ratio of women’s wages to men’s. Early on in the life course, women’s wages are 94% (ages 16-24) that of men’s. However, pay diverges over the lifespan, with a major expansion in the gender gap. At ages 25-34, women’s pay is 89% of men’s pay. By ages 35-44 women’s pay is only 83% of men’s, dropping to 80% at ages 45-54. Two key drivers for the gap expansion are differences in career interruptions and differences in weekly hours—both largely associated with motherhood.[30] In contrast, when men become parents, they tend to receive a premium, an increase in pay, rather than a penalty.[31] As of 2010, differences in human capital contributed little to the gender wage gap. However, differences in occupations were still important, as women tended to be in traditionally female occupations that are generally lower paying, such as nursing and teaching.[32]
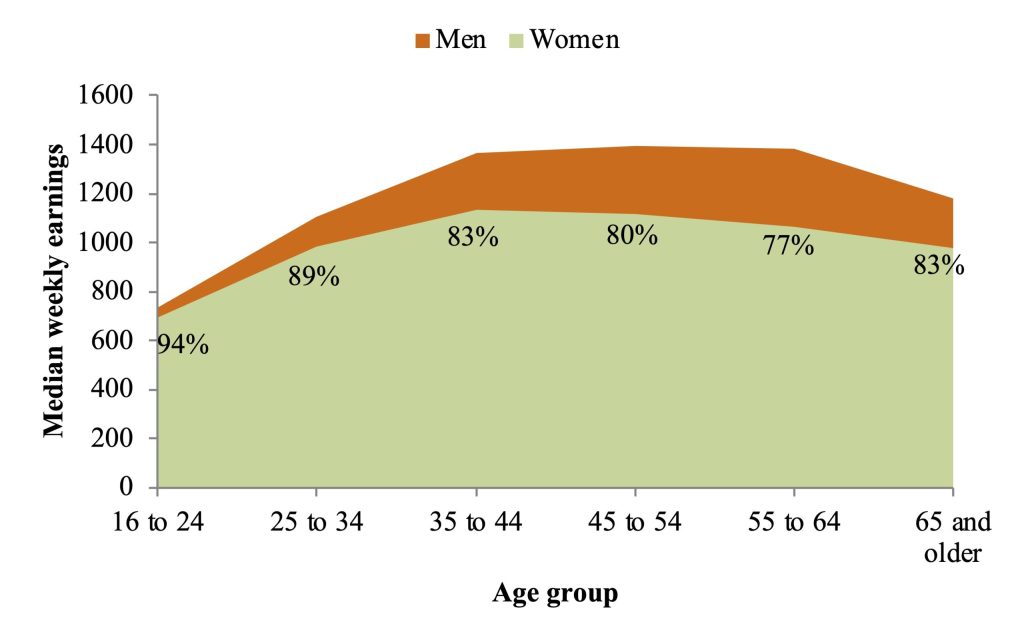
Gender pay gaps can be compounded by racial disparities. Figure 6.3[33] shows, for 2023, median weekly earnings among full-time workers as a percentage of white men’s earnings. People of color tend to earn less than whites, with disparities further exacerbated by the gender pay gap. For instance, Black men earn 79% of what white men earn and Hispanic men earn 75% of what white men earn. Asian men earn more than white men, at 133% and Asian women earn 106% of what white men earn. In contrast, other groups of women earn less on average. Black women earn 73% of what white men do and Hispanic women 65%. Relative to white women, who earn 83% of what white men earn, Asian women are better off but still at a disadvantage when compared to the relatively higher earnings of Asian men. Particularly concerningly, a study comparing the extent of racial and ethnic discrimination from 1969 to 2017 across Canada, France, Germany, Great Britain, the Netherlands, and the United States demonstrated that discrimination had not fallen over time, except slightly in France, and had even increased in the Netherlands.[34]
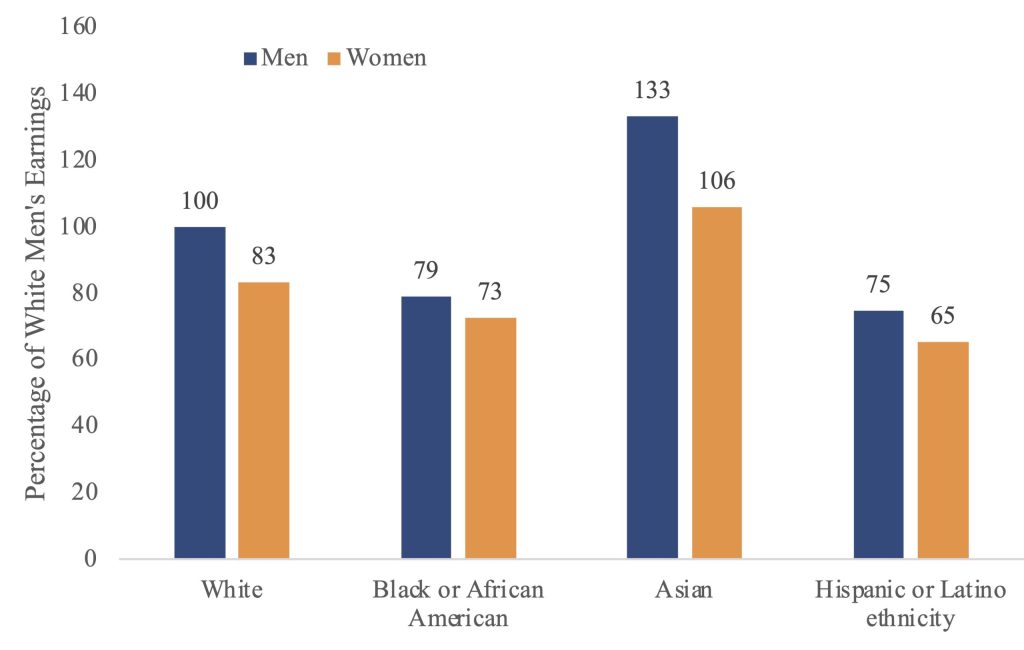
In studying pay gaps by race, what are referred to as pre-market factors, such as human capital, explain an important share of pay gaps. However, an important share of gaps are also discrimination in the labor market—estimated to be at least one-third of the Black-white wage gap.[35] Discrimination feeding into pay gaps can occur in complex ways. For example, when Black job-seekers attempt to negotiate for a higher salary, they are penalized in terms of their salary outcomes.[36] Likewise, women tend to be perceived more negatively than men when they try to negotiate, in part due to gender stereotypes around being “nice.”[37]
In the criminal justice system
Discrimination is a challenge throughout the criminal justice system and contributes to the large disparities in incarceration by race and gender that were discussed in the crime chapter.[38] Racial disparities in drug arrests are not due to differential drug or nondrug offending, nor residing in areas with a police focus on drug offenses; there is strong evidence of discrimination and disparities in police practices driving disparities.[39] Police officers in Chicago were more likely to ticket Black motorists for speeding than traffic cameras, suggesting racial bias in traffic enforcement.[40] Likewise, studies using the differential ability to tell driver race in the daytime versus the nighttime have demonstrated racial bias in traffic stops in some localities, but not others.[41] Once arrested, individuals may be discriminated against in terms of the process from pre-trial processing (for instance, setting bail) through setting their sentences.[42] Offenders who are Black, male, less educated, and lower income receive longer sentences.[43]
Policies to reduce discrimination
Competition
The idea of taste-based discrimination has, historically, been linked with the idea that competition may play a key role in reducing discrimination. Consider a case where all workers are equally productive, but some employers have discriminatory tastes. The non-discriminating employers would be able to make a greater profit by hiring individuals who tend to be discriminated against but are equally productive. This idea would suggest that the solution to discrimination in any market is simply competition. However, empirical evidence suggests that, while competitive markets deter discrimination, firms that have market power exist and do discriminate.[44] Simply “waiting out” discrimination will not be effective. Other interventions are required.
Changing the available information
An important set of interventions to reduce discrimination focus on changing the available information about individuals. Interventions can remove markers of protected categories, such as gender and race, from the set of available information to reduce discrimination. For example, when symphony orchestras adopted blind auditions—where the candidate plays music behind a screen and is not visible to the hiring committee—this approach led to gender equity in hiring, increasing the proportion of women in symphony orchestras.[45] However, policies to remove all potentially revealing information are challenging to design, and employers may be resistant to their implementation. For instance, orchestras have to lay down carpet, to muffle the sounds of heeled shoes that are associated with women, or ask women to take off their shoes.
Removing names from the available information may reduce discrimination in a variety of areas. This approach can be particularly effective for reducing discrimination in models like Uber and Lyft[46] or Airbnb[47] where such information could be readily removed without interrupting transactions. Other approaches to removing potential markers of protected categories include anonymizing resumes and using skills-based tests (like the orchestra auditions) for other jobs as well. A number of European countries have experimented with anonymizing applications.[48] Doing so can reduce disparities and equalize the probability of receiving an interview. However, the process still allows for discrimination in hiring after the interview and precludes affirmative actions for otherwise equivalent applicants. In France, anonymous resumes ultimately led to a lower probability of interviewing and hiring minority candidates.[49]
Depending on the nature of discrimination, there may be cases where removing information could be potentially harmful and adding information may be more helpful. One of the studies that identified discrimination in Airbnb determined that discrimination against African-American names disappeared when there was a positive public review.[50] Essentially, positive information about individuals helped reduce discrimination. Having to report gender-disaggregated information about pay has been shown to reduce the gender pay gap.[51] Providing gender-equitable guidance to managers can also lead them to switch from gender-inequitable to equitable raises.[52] However, having individuals disclose their past salaries when applying to new jobs can perpetuate discrimination, as new employers will use those as a basis for salary offers. This problem has led some states to ban asking applicants about their salary history.[53]
Box 6.2: The Lilly Ledbetter Fair Pay Act[54]
Lilly Ledbetter was an area manager for Goodyear from 1979 until 1998. Initially Ledbetter was paid the same as the men in the same position. By 1997, Ledbetter was paid $3,727 per month. Male managers were paid between $4,286 and $5,236 per month. In part because Goodyear kept pay information confidential (as is common practice), Ledbetter did not find out about the pay disparity until long after the disparity had occurred. When she sued, under Title VII of the Civil Rights Act, a 5-4 decision in the case before the Supreme Court determined that she had not filed within the statute of limitations—the legal time frame for filing after discrimination occurs. The case treated the discrimination as the decision about her salary by her supervisor, some time ago, not the ongoing disparate paychecks, because the paychecks themselves did not have discriminatory intent, which is required under Title VII. The problem that faced Lilly Ledbetter, that she learned about discrimination long after it occurred and the statute of limitations expired, led to the Lilly Ledbetter Fair Pay Act. The Act, passed in 2009, broadened the definition of discriminatory practice to include, for instance, each disparate paycheck. The case and subsequent act illustrate some of the challenges in identifying and remedying discriminatory practices.
The “Ban the box” campaign is an example of an information removal effort that appears to have achieved the opposite of its goal. We learned in the chapter on crime that ex-offender rehabilitation depends in part on employment opportunities and holding a legitimate job. Yet employers tend to discriminate against those with a criminal record.[55] Employers commonly ask about past criminal convictions on initial job applications. “Ban the box” campaigns forbid asking at the initial job application stage but allow for the question in interviews and with conditional job offers. The goal was that ex-offenders would have better job opportunities. An additional goal was to reduce racial disparities and discrimination in employment, given racial disparities and discrimination in the criminal justice system.[56] Although well intentioned, “ban the box” laws appear to be counterproductive in reducing discrimination. Employers, without information on criminal history, operate under statistical discrimination and are less likely to interview young, low-skilled Black and Hispanic men.[57]
Affirmative action
Affirmative action is a “set of procedures designed to eliminate unlawful discrimination between applicants, remedy the results of such prior discrimination, and prevent such discrimination in the future. Applicants may be seeking admission to an educational program or looking for professional employment.”[58] Affirmative action in the United States came about as a 1961 executive order by President John F. Kennedy, with a requirement mandating affirmative action among government contractors. Affirmative action subsequently expanded to other areas, such as education.
Those in favor of affirmative action argue that it equalizes opportunities, benefits qualified women and minorities, and that it is beneficial to society as a whole. Proponents also suggest that affirmative action improves equity and either improves efficiency or has at most minor reductions in efficiency through the reallocation of jobs. Opponents suggest that there are efficiency losses and that the policy itself is inherently racist.[59]
Historically, affirmative action has helped promote the employment of minorities and women.[60] The magnitude of the effects is generally fairly small, although they can cause substantial relative shifts for minority groups.[61] While minorities who benefit on the labor market may have poorer credentials, they have equal performance, suggesting that efficiency concerns have relatively little merit. Further, white males face costs, but they are relatively small. Affirmative action has also increased the probability that under-represented minority groups graduate from selective institutions. However, affirmative action or some approaches to affirmative action have been banned in making university admissions decisions.[62]
Reducing bias in individuals
Individuals’ biases, for example their gender biases, are key drivers of discrimination.[63] Legal changes can potentially change individuals’ attitudes and behavior. For example, the passage of same-sex marriage reforms in U.S. states reduced individuals’ discrimination against sexual minorities. This reduced discrimination in turn contributed to improvements in labor market outcomes for same-sex couples.[64]
Individuals may not be aware of their biases, in which case they are referred to as implicit biases. Policies and practices that increase interaction with individuals from groups that are discriminated against have been shown to reduce such biases.[65] Training can also reduce implicit biases, particularly those that may be caused by lack of exposure or familiarity with other races.[66] Treating implicit bias like a habit that can be combated through awareness, concern about its effects, and the use of strategies to reduce bias is helpful, particularly for people who are concerned about discrimination in the first place.[67] This suggests that training to reduce bias in individuals requires some commitment on their part to change their thinking and behaviors, and therefore is likely to work better for some individuals and biases than others.
Professionalizing human resources functions may also help reduce bias in the hiring process. Research in Canada demonstrated that employers discriminated against those with Asian-sounding names. Asian applicants had a 20% disadvantage for large employers but double the disadvantage, 40%, for small employers. Larger organizations may devote more resources to recruitment, have professional human resource strategies, and also have more experience with diverse staff.[68] This professionalism may reduce (although not necessarily eliminate) discrimination.
It may even be possible to reduce the role of biased human decision making in areas such as sentencing. Risk assessments are a potential, but controversial, approach to reducing bias in sentencing, parole, and rehabilitation.[69] Risk assessment instruments model the probability of reoffending based on a number of factors, including criminal history. In part because of different criminal histories, the policy can have disparate impact across racial groups. For example, Black offenders receive higher risk assessments, on average, than white offenders.[70] Especially with disparities in the criminal justice system, such instruments may perpetuate disparities. However, improvements in computing, such as machine learning algorithms, have the potential to reduce jail populations and crime rates, including reducing the percentage of minorities in jail.[71] Yet machine learning and artificial intelligence can also pick up and replicate existing biases.[72]
Conclusions
Discrimination occurs in education, employment, housing, and the criminal justice system, as well as many other dimensions of individuals’ lives. Economists tend to understand discrimination through one of two models—based on prejudicial “tastes” for discrimination or based on incomplete information leading to statistical discrimination. Both theories of discrimination show how discrimination contributes to disparate outcomes, such as different wages and employment rates for men and women. Although discrimination is pervasive, the good news is that progress is being made, and (some) disparities have decreased over time as a result of effective policies. Designing effective policies is, however, extremely challenging, as the counter-productive efforts to “ban the box” illustrate. The challenges of designing effective policies underline an important role for economists and statisticians in the fight against discrimination: carefully evaluating the impact of different policy and program attempts to reduce discrimination.
List of terms
- Discrimination
- Disparities
- Taste-driven discrimination
- Statistical discrimination
- Marginal revenue product
- Multiple regression
- Audit studies
- Affirmative action
- Implicit biases
References
Abbott Watkins, Torie. “The Ghost of Salary Past: Why Salary History Inquiries Perpetuate the Gender Pay Gap and Should Be Ousted as a Factor Other Than Sex.” Minnesota Law Review 69, no. 1041–1088 (2018).
Agan, Amanda, and Sonja Starr. “Ban the Box, Criminal Records, and Racial Discrimination: A Field Experiment.” The Quarterly Journal of Economics 133, no. 1 (2018): 191–235. doi:10.1093/qje/qjx028.Advance.
Ahmed, Ali M., and Mats Hammarstedt. “Discrimination in the Rental Housing Market: A Field Experiment on the Internet.” Journal of Urban Economics 64, no. 2 (2008): 362–72. doi:10.1016/j.jue.2008.02.004.
Alesina, Alberto F., Michela Carlana, Eliana La Ferrara, and Paolo Pinotti. “Revealing Stereotypes: Evidence from Immigrants in Schools.” American Economic Review 114, no. 7 (2024): 1916–48.
Alfitian, Jakob, Marvin Deversi, and Dirk Sliwka. “Closing the Gender Gap in Salary Increases: Evidence from a Field Experiment on Promoting Pay Equity.” IZA Discussion Paper Series, 2023.
Antonovics, Kate, and Brian G. Knight. “A New Look at Racial Profiling: Evidence from the Boston Police Department.” Review of Economics and Statistics 91, no. 1 (2009): 163–77. doi:10.1162/rest.91.1.163.
Bailey, Martha J., Tanya S. Byker, Elena Patel, and Shanthi Ramnath. “The Long-Term Effects of California’s 2004 Paid Family Leave Act on Women’s Careers: Evidence from U.S. Tax Data.” NBER Working Paper Series. Cambridge, MA, 2019.
Banerjee, Rupa, Jeffrey G. Reitz, and Philip Oreopoulos. “Do Large Employers Treat Racial Minorities More Fairly? A New Analysis of Canadian Field Experiment Data.” University of Toronto Robert F. Harney Program in Ethnic, Immigration, and Pluralism Studies, 2017.
Battaglini, Marco, Jorgen M. Harris, and Eleonora Patacchini. “Interactions with Powerful Female Colleagues Promote Diversity in Hiring.” Journal of Labor Economics 41, no. 3 (2023): 589–614.
Becker, Gary S. The Economics of Discrimination. Chicago, IL: University of Chicago Press, 1971.
Behaghel, Luc, Bruno Crépon, and Thomas Le Barbanchon. “Unintended Effects of Anonymous Résumés.” American Economic Journal: Applied Economics 7, no. 3 (2015): 1–27. doi:10.1257/app.20140185.
Bellemare, Charles, Marion Gousse, Guy Lacroix, and Steeve Marchand. “Physical Disability and Labor Market Discrimination: Evidence from a Field Experiment.” HCEO Working Paper Series, 2018.
Bennedsen, Morten, Elena Simintzi, Margarita Tsoutsoura, and Daniel Wolfenzon. “Do Firms Respond to Gender Pay Gap Transparency?” NBER Working Paper Series. Cambridge, MA, 2018.
Bertrand, Marianne, Claudia Goldin, and Lawrence F. Katz. “Dynamics of the Gender Gap for Young Professionals in the Financial and Corporate Sectors.” American Economic Journal: Applied Economics 2, no. 3 (2010): 228–55.
Bertrand, Marianne, and Sendhil Mullainathan. “Are Emily and Greg More Employable Than Lakisha and Jamal? A Field Experiment on Labor Market Discrimination.” American Economic Review 94, no. 4 (2004): 991–1013. doi:10.1257/0002828042002561.
Bielen, Samantha, Wim Marneffe, and Naci H. Mocan. “Racial Bias and In-Group Bias in Judicial Decisions: Evidence from Virtual Reality Courtrooms.” NBER Working Paper Series. Cambridge, MA, 2018.
Blau, Francine D., and Lawrence M. Kahn. “The Gender Wage Gap: Extent, Trends, and Explanations.” National Bureau of Economic Research Working Paper Series, 2016. doi:10.1007/s10273-011-1262-2.
Board of Governors of the Federal Reserve. “Board Members: Lisa D. Cook,” 2022. https://www.federalreserve.gov/aboutthefed/bios/board/cook.htm.
Bonczar, Thomas P. “Prevalence of Imprisonment in the U.S. Population, 1974-2001.” Bureau of Justice Statistics Special Report, 2003.
Bowles, Hannah R., Linda Babcock, and Lei Lai. “Social Incentives for Gender Differences in the Propensity to Initiate Negotiations: Sometimes It Does Hurt to Ask.” Organizational Behavior and Human Decision Processes 103 (2007): 84–103. doi:10.1016/j.obhdp.2006.09.001.
Caliskan, Aylin, Joanna J. Bryson, and Arvind Narayanan. “Semantics Derived Automatically from Language Corpora Contain Human-like Biases.” Science 356 (2017): 183–86.
Carruthers, Celeste K., and Marianne H. Wanamaker. “Separate and Unequal in the Labor Market: Human Capital and the Jim Crow Wage Gap.” Journal of Labor Economics 35, no. 3 (2017): 655–96.
Cook, Lisa D. “Biographical Information for Lisa D. Cook, Ph.D.” Lisadcook.Net, 2019. https://lisadcook.net/wp-content/uploads/2020/02/cook_web_bio_revised_1219_rev-1.pdf.
———. “Converging to a National Lynching Database: Recent Developments.” Michigan State University, 2011.
Cook, Lisa D., Maggie E. C. Jones, David Rose, and Trevon D. Logan. “The Green Books and the Geography of Segregation in Public Accomodations.” NBER Working Paper Series. Cambridge, MA, 2020.
Cornell University Law School. “Affirmative Action.” Wex Legal Dictionary, 2017. https://www.law.cornell.edu/wex/affirmative_action.
Corno, Lucia, Eliana La Ferrara, and Justine Burns. “Interaction, Stereotypes, and Performance: Evidence from South Africa.” American Economic Review 112, no. 12 (2022): 3848–75.
Cui, Ruomeng, Jun Li, and Dennis J. Zhang. “Discrimination with Incomplete Information in the Sharing Economy: Field Evidence from Airbnb,” 2016.
Cullen, Zoë, and Ricardo Perez-Truglia. “The Old Boys’ Club: Schmoozing and the Gender Gap.” American Economic Review 113, no. 7 (2023): 1703–40.
Datta, Saugato, and Vikram Pathania. “For Whom Does the Phone (Not) Ring? Discrimination in the Rental Housing Market in India.” WIDER Working Paper, 2016.
Desmarais, Sarah L., and Jay P. Singh. “Risk Assessment Instruments Validated and Implemented in Correctional Settings in the United States,” 2013.
Devine, Patricia G., Patrick S. Forscher, Anthony J. Austin, and William T. L. Cox. “Long-Term Reduction in Implicit Race Bias: A Prejudice Habit-Breaking Intervention.” Journal of Experimental Social Psychology 48, no. 6 (2012): 1267–78. doi:10.1016/j.jesp.2012.06.003.
Doleac, Jennifer L. “Racial Bias in the Criminal Justice System.” In A Modern Guide to the Economics of Crime, edited by Paolo Buonnano, Paolo Vanin, and Juan Vargas, 286–304. Edward Elgar Publishing, 2022. doi:10.4337/9781789909333.00017.
Doleac, Jennifer L., and Benjamin Hansen. “Does ‘Ban the Box’ Help or Hurt Low-Skilled Workers? Statistical Discrimination and Employment Outcomes When Criminal Histories Are Hidden.” NBER Working Paper Series. Cambridge, MA, 2016.
Edelman, Benjamin, Michael Luca, and Svirsky Dan. “Racial Discrimination in the Sharing Economy: Evidence from a Field Experiment.” American Economic Journal: Applied Economics 9, no. 2 (2017): 1–22. doi:10.2139/ssrn.2701902.
Ewens, Michael, Bryan Tomlin, and Liang Choon Wang. “Statistical Discrimination or Prejudice? A Large Sample Field Experiment.” The Review of Economics and Statistics 96, no. 1 (2014): 119–34. doi:10.2139/ssrn.1816702.
Ewijk, Reyn Van. “Same Work, Lower Grade? Student Ethnicity and Teachers’ Subjective Assessments.” Economics of Education Review 30, no. 5 (2011): 1045–58. doi:10.1016/j.econedurev.2011.05.008.
Fryer Jr., Roland G., Devah Pager, and Jörg L. Spenkuch. “Racial Disparities in Job Finding and Offered Wages.” Journal of Law & Economics 56, no. 3 (2013): 633–89. doi:10.1086/673323.
Ge, Yanbo, Christopher R. Knittel, Don Mackenzie, and Stephen Zoepf. “Racial and Gender Discrimination in Transportation Network Companies.” NBER Working Paper Series, 2016. http://www.nber.org/papers/w22776.
Goldin, Claudia, Sari Pekkala Kerr, and Claudia Olivetti. “The Other Side of the Mountain: Women’s Employment and Earnings over the Family Cycle.” Oxford Open Economics 3, no. Supplement_1 (2024): i323–34. doi:10.1093/ooec/odad012.
Goldin, Claudia, and Cecilia Rouse. “Orchestrating Impartiality: The Impact of ‘Blind’ Auditions on Female Musicians.” The American Economic Review 90, no. 4 (2000): 715–41. doi:10.1257/aer.90.4.715.
Hellerstein, Judith K., David Neumark, and Kenneth R. Troske. “Market Forces and Sex Discrimination.” Journal of Human Resources 37, no. 2 (2002): 353–80. doi:10.3386/w6321.
Henry, Jessica S., and James B. Jacobs. “Ban the Box to Promote Ex-Offender Employment.” Criminology and Public Policy 6, no. 4 (2007): 755–62. doi:10.1111/j.1745-9133.2007.00470.x.
Hernandez, Morela, Derek R. Avery, Sabrina D. Volpone, and Cheryl R. Kaiser. “Bargaining While Black: The Role of Race in Salary Negotiations.” Journal of Applied Psychology 104, no. 4 (2019): 581–92.
Hinrichs, Peter. “The Effects of the National School Lunch Program on Education and Health.” Journal of Policy Analysis and Management 29, no. 3 (2010): 479–505. doi:10.1002/pam.20506.
Holzer, Harry J., and David Neumark. “Affirmative Action: What Do We Know?” Journal of Policy Analysis and Management 25, no. 2 (2006): 463–90.
Ibanez, Marcela, and Gerhard Riener. “Sorting through Affirmative Action: Three Field Experiments in Colombia.” Journal of Labor Economics 36, no. 2 (2018): 437–78.
Jones, Melanie K., Ezgi Kaya, and Kerry L. Papps. “The Ongoing Impact of Gender Pay Gap Transparency Legislation.” IZA Discussion Paper Series, 2022.
Kleinberg, Jon, Himabindu Lakkaraju, Jure Leskovec, Jens Ludwig, and Sendhil Mullainathan. “Human Decisions and Machine Predictions.” NBER Working Paper, 2017. doi:10.3386/w23180.
Krause, Annabelle, Ulf Rinne, and Klaus F. Zimmermann. “Anonymous Job Applications in Europe.” IZA Journal of European Labor Studies 1, no. 5 (2012).
Laouénan, Morgane, and Roland Rathelot. “Can Information Reduce Ethnic Discrimination? Evidence from Airbnb.” American Economic Journal: Applied Economics 14, no. 1 (2022): 107–32.
Lebrecht, Sophie, Lara J. Pierce, Michael J. Tarr, and James W. Tanaka. “Perceptual Other-Race Training Reduces Implicit Racial Bias.” PLoS ONE 4, no. 1 (2009). doi:10.1371/journal.pone.0004215.
Leonard, Jonathan S. “The Impact of Affirmative Action Regulation and Equal Employment Law on Black Employment.” Journal of Economic Perspectives 4, no. 4 (1990): 47–63.
Lundberg, Shelly, and Elaina Rose. “The Effects of Sons and Daughters on Men’s Labor Supply and Wages.” The Review of Economcis and Statistics 84, no. 2 (2002): 251–68.
Mitchell, Ojmarrh, and Michael S. Caudy. “Examining Racial Disparities in Drug Arrests.” Justice Quarterly 32, no. 2 (2015): 288–313. doi:10.1080/07418825.2012.761721.
Moss-Racusin, Corinne A., John F. Dovidio, Victoria L. Brescoll, Mark J. Graham, and Jo Handelsman. “Science Faculty’s Subtle Gender Biases Favor Male Students.” Proceedings of the National Academy of Sciences of the United States of America 109, no. 41 (October 9, 2012): 16474–79. doi:10.1073/pnas.1211286109.
Mustard, David B. “Racial, Ethnic, and Gender Disparities in Sentencing: Evidence from the U.S. Federal Courts.” The Journal of Law & Economics 44, no. 1 (2001): 285–314.
Neumark, David, Roy J. Bank, and Kyle D. Van Nort. “Sex Discrimination in Restaurant Hiring: An Audit Study.” The Quarterly Journal of Economics 111, no. 3 (1996): 915–41.
Oreopoulos, Philip. “Why Do Skilled Immigrants Struggle in the Labor Market? A Field Experiment with Thirteen Thousand Resumes.” American Economic Journal: Economic Policy 3, no. 4 (2011): 148–71. doi:10.1257/pol.3.4.148.
Pager, Devah. “The Mark of a Criminal Record.” American Journal of Sociology 108, no. 5 (2003): 937–75.
Pager, Devah, Bruce Western, and Bart Bonikowsi. “Discrimination in a Low-Wage Labor Market: A Field Experiment.” American Sociological Review 74, no. 5 (2009): 777–99. doi:10.1177/000312240907400505.Discrimination.
Phelps, Edmund S. “The Statistical Theory of Racism and Sexism.” American Economic Review 62, no. 4 (1972): 659–61.
Quillian, Lincoln, and John J. Lee. “Trends in Racial and Ethnic Discrimination in Hiring in Six Western Countries.” Proceedings of the National Academy of Sciences 120, no. 6 (2023): e2212875120. doi:10.1073/pnas.
Ritter, Joseph, and David Bael. “Detecting Racial Profiling in Minneapolis Traffic Stops: A New Approach.” CURA Reporter Summer/Spr (2009): 11–17.
Sansone, Dario. “Pink Work: Same-Sex Marriage, Employment and Discrimination.” Journal of Public Economics 180 (2019): 104086. doi:10.1016/j.jpubeco.2019.104086.
Schlesinger, Traci. “Racial and Ethnic Disparity in Pretrial Criminal Processing.” Justice Quarterly 22, no. 2 (2007): 170–92.
Skeem, Jennifer, and Christopher T. Lowenkamp. “Risk, Race, & Recidivism: Predictive Bias and Disparate Impact.” Crimonology 54, no. 4 (2016): 680–712.
Sorock, Carolyn E. “Closing the Gap Legislatively: Consequences of the Lilly Ledbetter Fair Pay Act.” Chicago-Kent Law Review 85, no. 3 (2010): 1199–1216.
Sprietsma, Maresa. “Discrimination in Grading: Experimental Evidence from Primary School Teachers.” Empirical Economics 45, no. 1 (2013): 523–38. doi:10.1007/s00181-012-0609-x.
Starr, Sonja B., and M. Marit Rehavi. “Mandatory Sentencing and Racial Disparity: Assessing the Role of Prosecutors and the Effects of Booker.” Yale Law Journal 123, no. 1 (2013): 2–80. doi:10.1525/sp.2007.54.1.23.
U.S. Bureau of Labor Statistics. “Highlights of Women’s Earnings in 2023.” BLS Reports Report 1111, 2024.
U.S. Equal Employment Opportunity Commission. “Discrimination by Type,” 2017. https://www.eeoc.gov/laws/types/.
UN Women. “Convention on the Elimination of All Forms of Discrimination against Women,” 2009. http://www.un.org/womenwatch/daw/cedaw/text/econvention.htm.
United Nations Human Rights Office of the High Commissioner. “International Convention on the Elimination of All Forms of Racial Discrimination,” 2017. http://www.ohchr.org/EN/ProfessionalInterest/Pages/CERD.aspx.
Weichselbaumer, Doris. “Multiple Discrimination against Female Immigrants Wearing Headscarves.” ILR Review, 2019, 1–28. doi:10.1177/0019793919875707.
Welty, Leah J., Anna J. Harrison, Karen M. Abram, Nichole D. Olson, David A. Aaby, Kathleen P. Mccoy, Jason J. Washburn, and Linda A. Teplin. “Health Disparities in Drug- and Alcohol-Use Disorders: A 12-Year Longitudinal Study of Youths After Detention.” American Journal of Public Health 106, no. 5 (2016): 872–80. doi:10.2105/AJPH.2015.303032.
Xu, Wenfei, Michael Smart, Nebiyou Tilahun, Sajad Askari, Zachary Dennis, Houpu Li, and David Levinson. “The Racial Composition of Road Users, Traffic Citations, and Police Stops.” Proceedings of the National Academy of Sciences of the United States of America 121, no. 24 (2024): 6–11. doi:10.1073/pnas.2402547121.
Yinger, John. “Measuring Racial Discrimination with Fair Housing Audits: Caught in the Act.” American Economic Review 76, no. 5 (1986): 881–93.
- U.S. Equal Employment Opportunity Commission, 2017. ↵
- UN Women, 2009; United Nations Human Rights Office of the High Commissioner, 2017. ↵
- U.S. Bureau of Labor Statistics, 2024. ↵
- Bonczar, 2003. ↵
- Doleac, 2022. ↵
- Becker, 1971. ↵
- Corno, La Ferrera, and Burns 2022. ↵
- Phelps, 1972. ↵
- Cook et al., 2020; Cook, 2011; Cook, 2019; Board of Governors of the Federal Reserve, 2022. ↵
- Hellerstein, Neumark, and Troske, 2002. ↵
- Carruthers and Wanamaker, 2017. ↵
- Sprietsma, 2013. ↵
- Van Ewijk, 2011. ↵
- Moss-Racusin et al., October 9, 2012. ↵
- Yinger, 1986. ↵
- Ibid. ↵
- Ewens, Tomlin, and Wang, 2014. ↵
- Datta and Pathania, 2016. ↵
- Ahmed and Hammarstedt, 2008. ↵
- Edelman, Luca, and Dan, 2017. ↵
- Laouénan and Rathelot, 2022. ↵
- Bertrand and Mullainathan, 2004. ↵
- Pager, Western, and Bonikowsi, 2009. ↵
- Neumark, Bank, and Van Nort, 1996. ↵
- Bellemare et al., 2018. ↵
- Oreopoulos, 2011. ↵
- Weichselbaumer, 2019. ↵
- U.S. Bureau of Labor Statistics, 2024. ↵
- Ibid. ↵
- Bertrand, Goldin, and Katz, 2010; Bailey et al., 2019; Goldin, Kerr, and Olivetti 2024. ↵
- Lundberg and Rose, 2002; Goldin, Kerr, and Olivetti 2024. ↵
- Blau and Kahn, 2016. ↵
- U.S. Bureau of Labor Statistics, 2024. ↵
- Quillian and Lee 2024. ↵
- Fryer Jr., Pager, and Spenkuch, 2013. ↵
- Hernandez et al., 2019. ↵
- Bowles, Babcock, and Lai, 2007. ↵
- Doleac, 2022. ↵
- Mitchell and Caudy, 2015; Welty et al., 2016. ↵
- Xu et al. 2024. ↵
- Ritter and Bael, 2009; Antonovics and Knight, 2009. ↵
- Schlesinger, 2007; Starr and Rehavi, 2013; Bielen, Marneffe, and Mocan, 2018. ↵
- Mustard, 2001; Cook et al., 2020. ↵
- Hellerstein, Neumark, and Troske, 2002. ↵
- Goldin and Rouse, 2000. ↵
- Ge et al., 2016. ↵
- Edelman, Luca, and Dan, 2017. ↵
- Krause, Rinne, and Zimmermann, 2012; Behaghel, Crépon, and Le Barbanchon, 2015. ↵
- Behaghel, Crépon, and Le Barbanchon, 2015. ↵
- Cui, Li, and Zhang, 2016. ↵
- Bennedsen et al., 2018; Jones, Kaya, and Papps, 2022. ↵
- Alfitian, Deversi, and Sliwka, 2023. ↵
- Abbott Watkins, 2018. ↵
- Sorock, 2010. ↵
- Pager, 2003. ↵
- Henry and Jacobs, 2007. ↵
- Doleac and Hansen, 2016; Agan and Starr, 2018. ↵
- Cornell University Law School, 2017. ↵
- Holzer and Neumark, 2006; Ibanez and Riener, 2018. ↵
- Leonard, 1990. ↵
- Holzer and Neumark, 2006. ↵
- Hinrichs, 2010. ↵
- E.g. Moss-Racusin et al., October 9, 2012. ↵
- Sansone, 2019. ↵
- Cullen and Perez-Truglia, 2023; Corno, La Ferrara, and Burns, 2022; Battaglini, Harris, and Patacchini, 2023. ↵
- Lebrecht et al., 2009. ↵
- Devine et al., 2012; Alesina et al., 2024. ↵
- Banerjee, Reitz, and Oreopoulos, 2017. ↵
- Desmarais and Singh, 2013. ↵
- Skeem and Lowenkamp, 2016. ↵
- Kleinberg et al., 2017. ↵
- Caliskan, Bryson, and Narayanan, 2017. ↵